Abstract
We present DNA-Rendering, a large-scale, high-fidelity repository of neural actor rendering represented by neural implicit fields of human actors. We provide human subjects with
- High Diversity: We collected a total of 500 individuals, with 527 different sets of clothing, 269 different types of daily actions, and 153 different types of special performances, including relevant interactive objects for some actions.
- High Fidelity: We construct professional multi-view system to capture data, which contains 60 synchronous cameras with max 4096 × 3000 resolution, 15fps speed.
- Rich Annotations: We provide off-the-shelf annotations including 2D/3D human body keypoints, foreground masks and SMPL-X models.
Core Features
IDxOutfit
Motions
Views
Frames
Max Resolution
Scales
To our knowledge, our dataset far surpasses similar ones in terms of the number of actors, costumes, actions, clarity, and overall data volume.
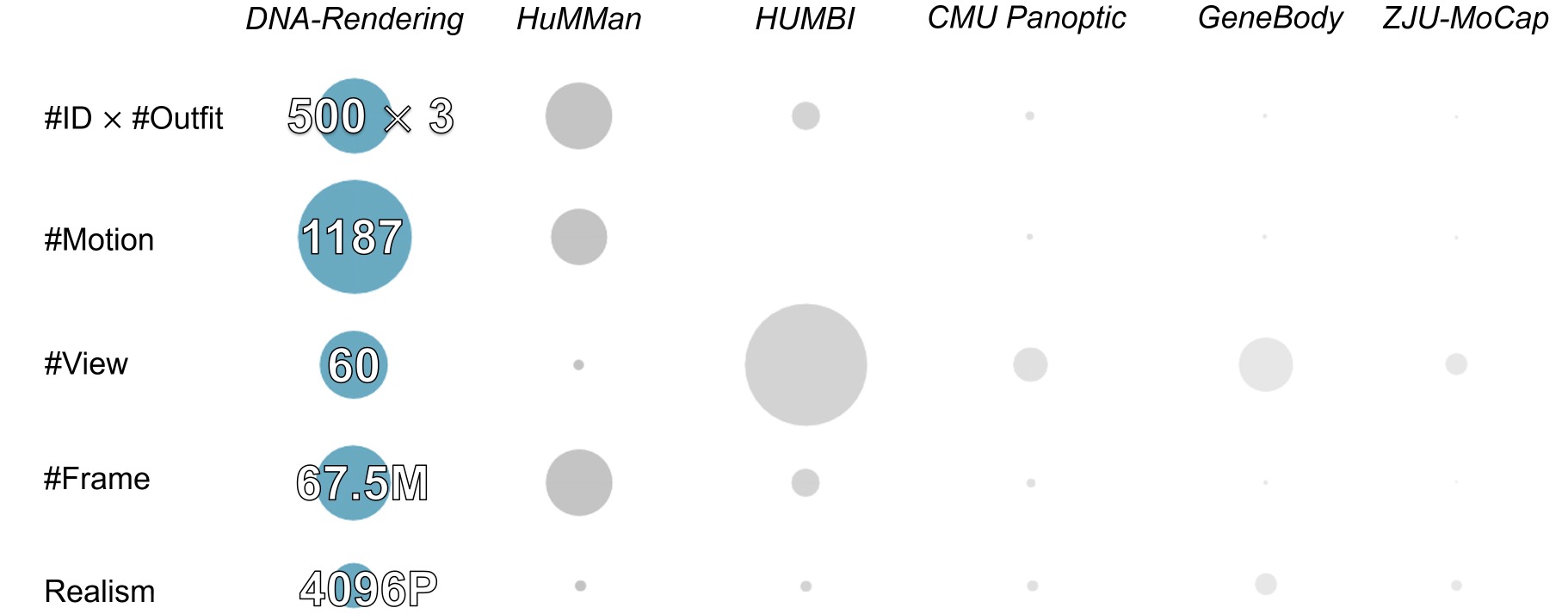
Diversity
Our dataset covers a diverse range of scenarios, including everyday and special performances. It includes a total of 527 clothing types and 422 action types, with sufficient difficulty levels in clothing textures and motion complexity. This diversity and difficulty make it suitable for a variety of downstream research tasks.
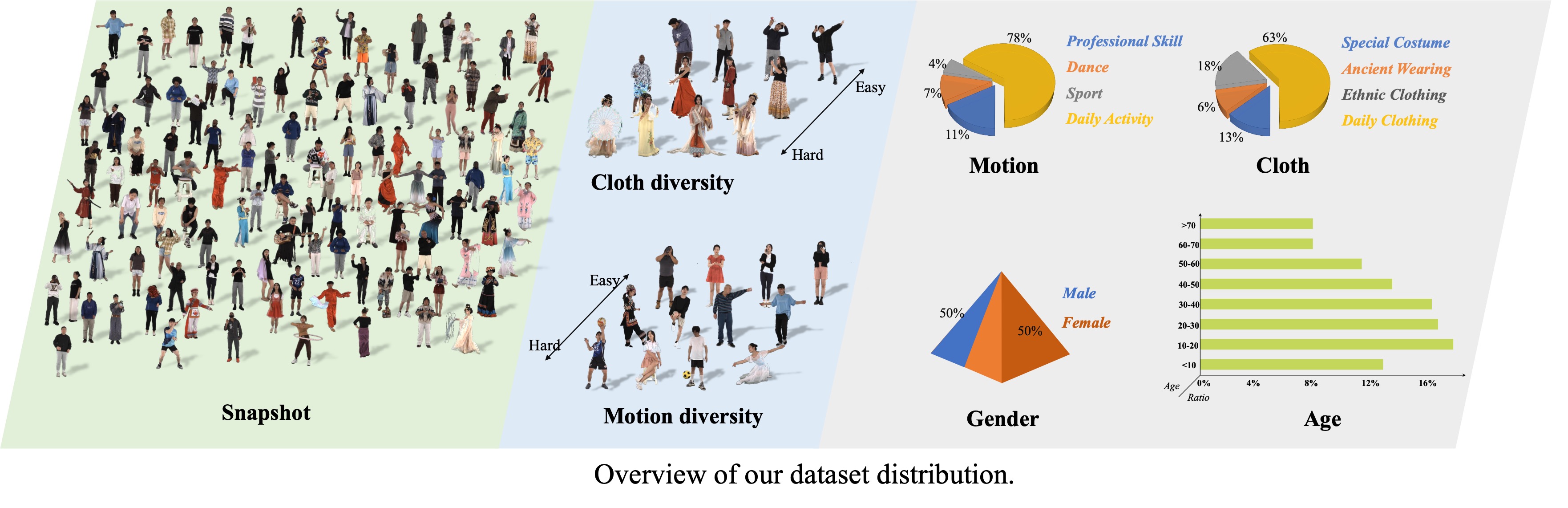
High-quality Annotations
Our dataset comes with off-the-shelf high-precision annotation, including 2D/3D human body keypoints, foreground masks, and SMPL-X models. We have specifically optimized our annotations for 3D human body scenarios, resulting in high-quality annotations.
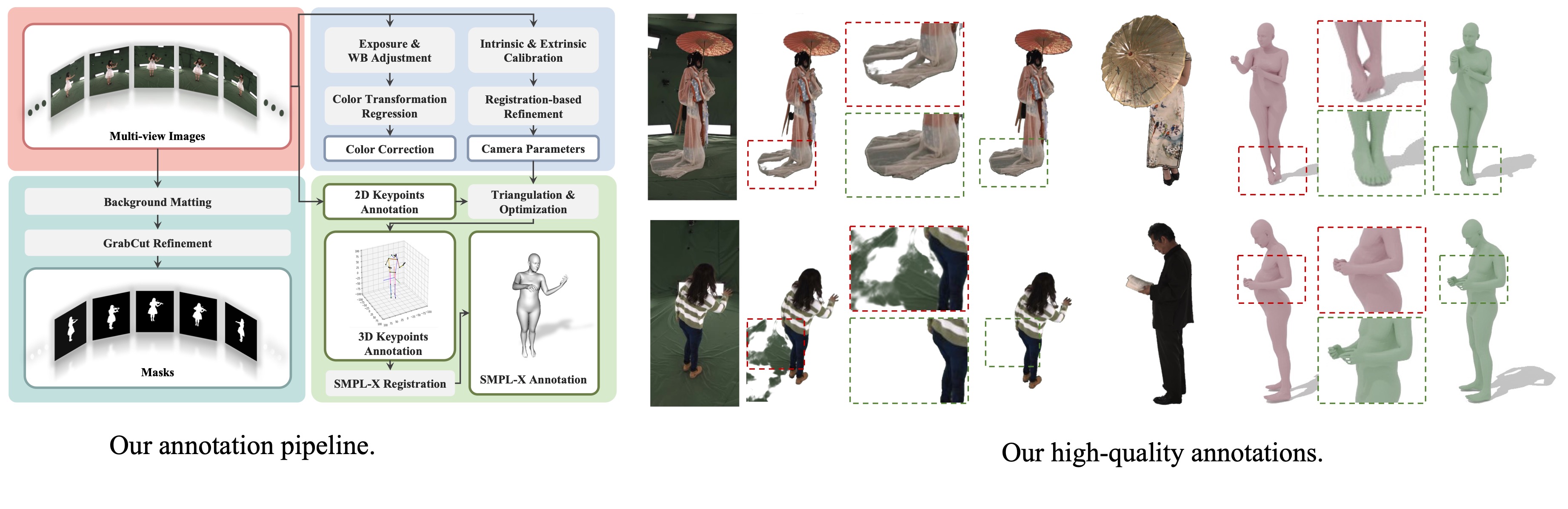
Benchmarks
We have provided the results of various state-of-the-art methods of rendering and animation on our dataset.
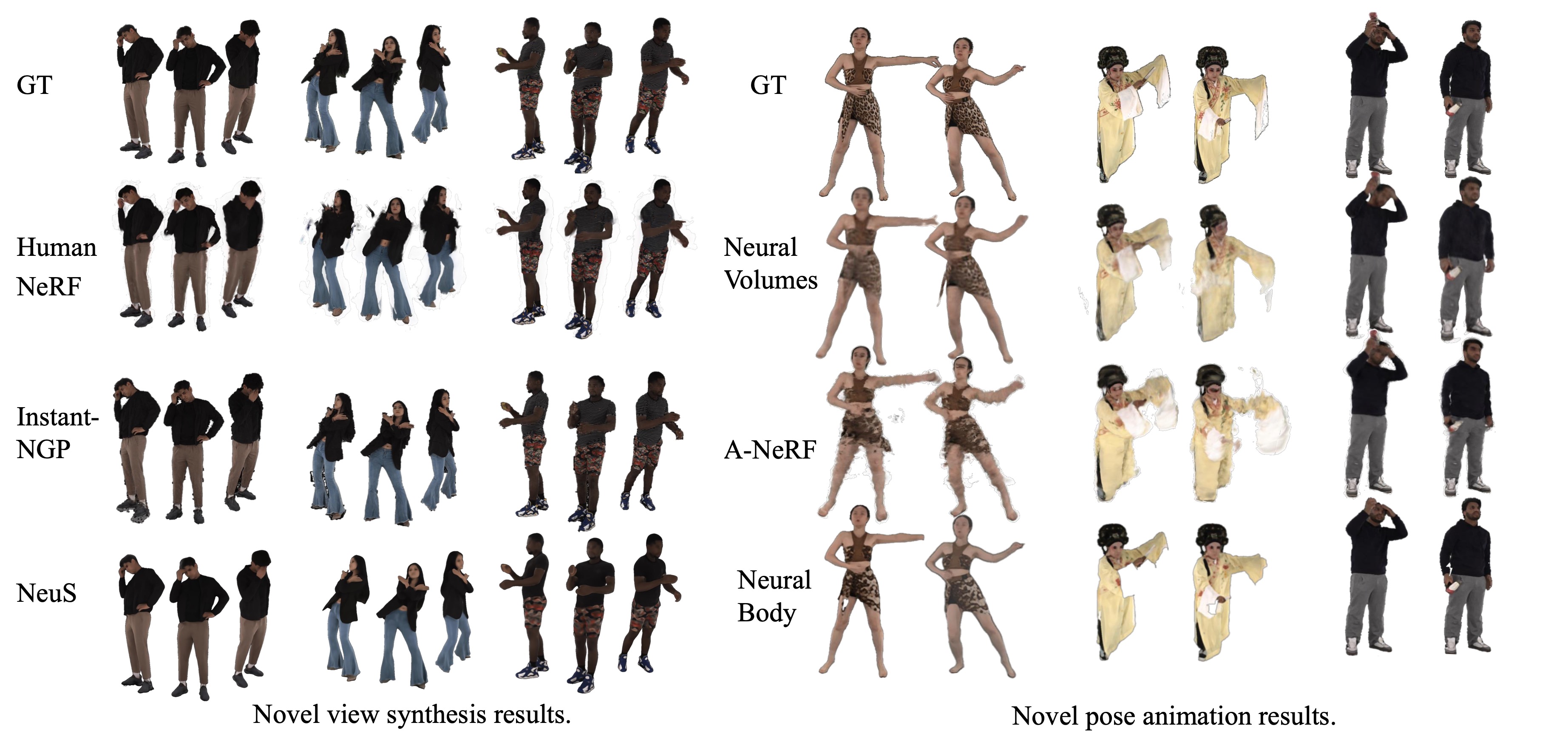
Contributors
The DNA-Rendering Team
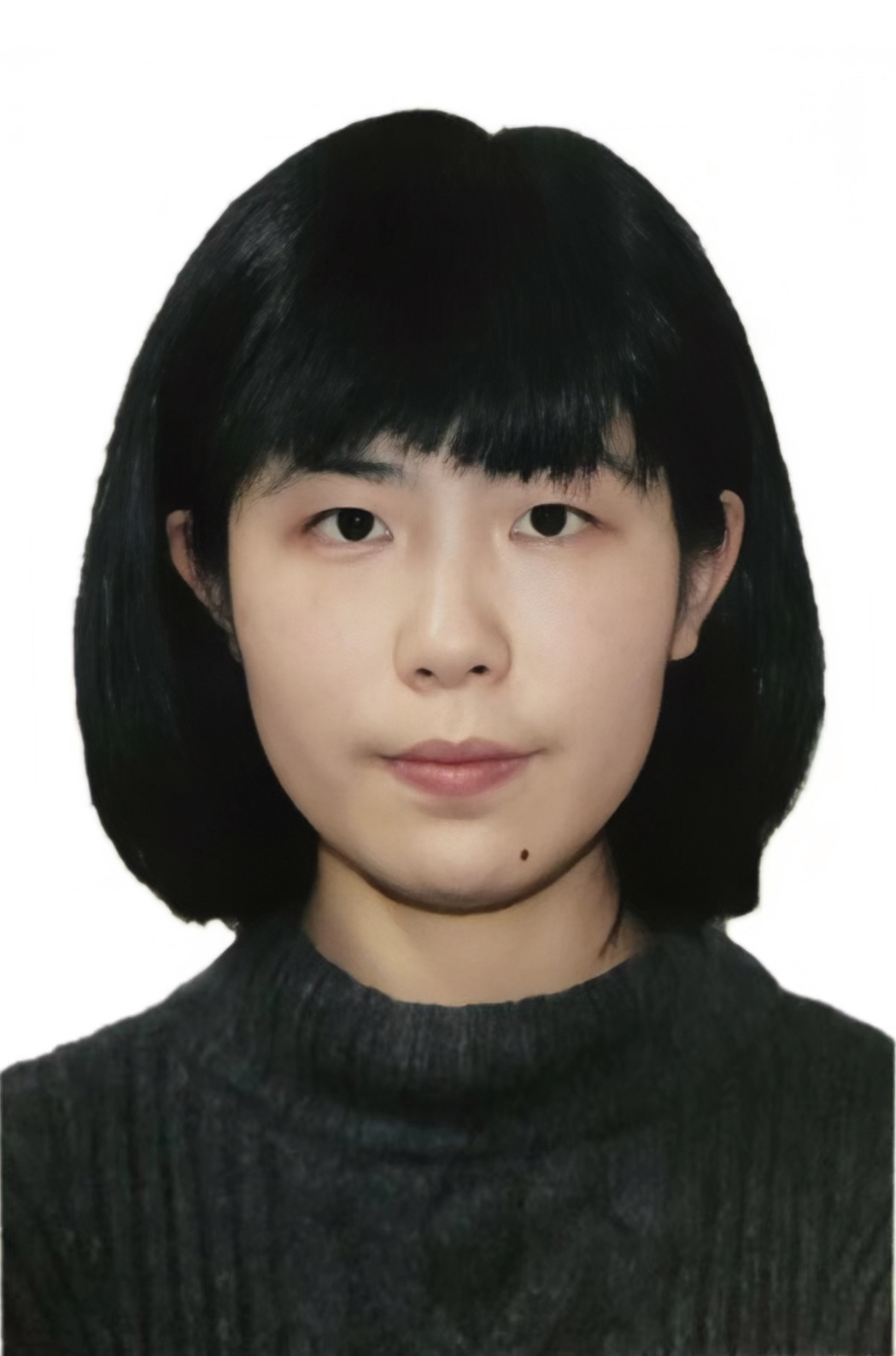
Huiwen Luo
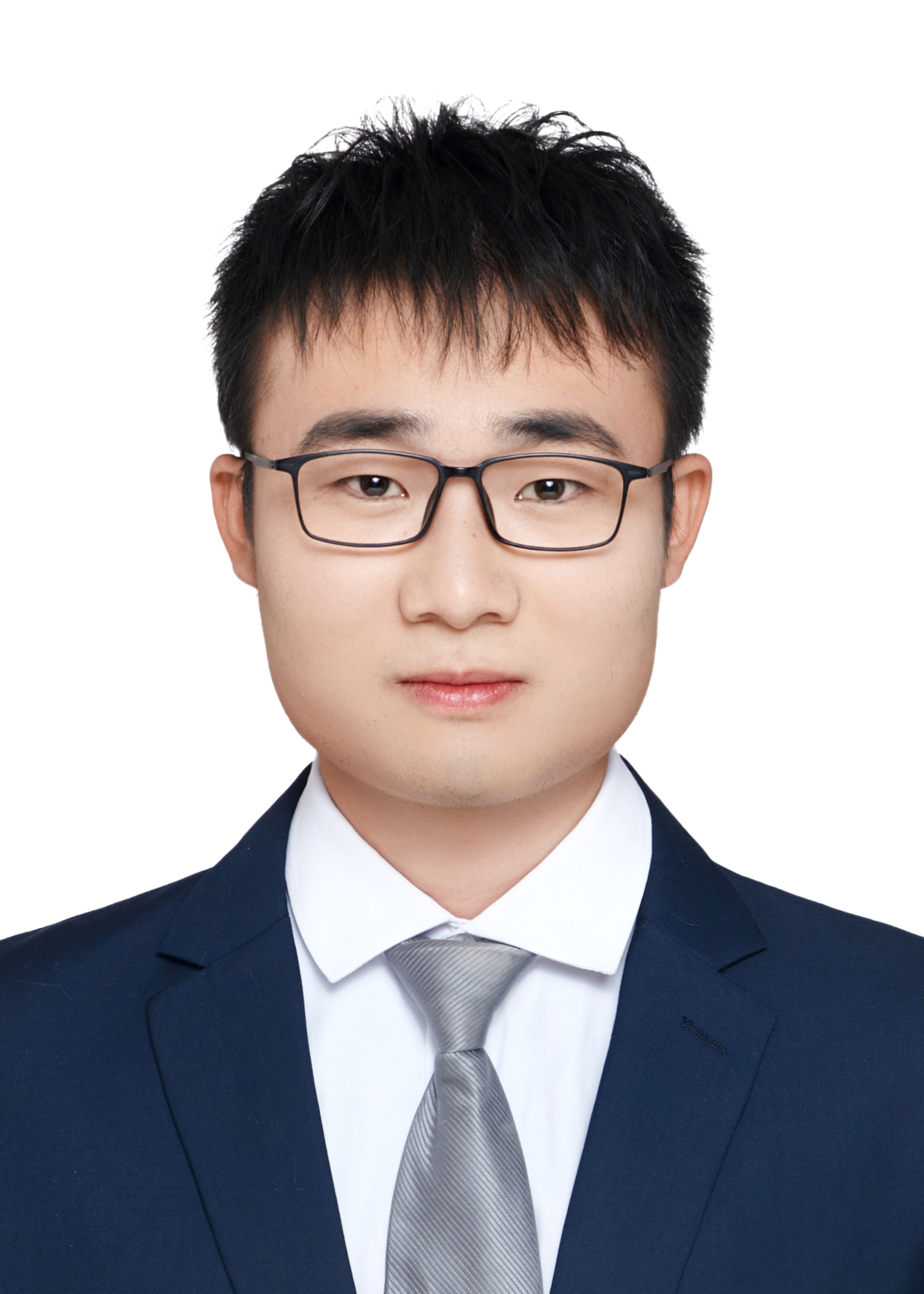
Fan Lu
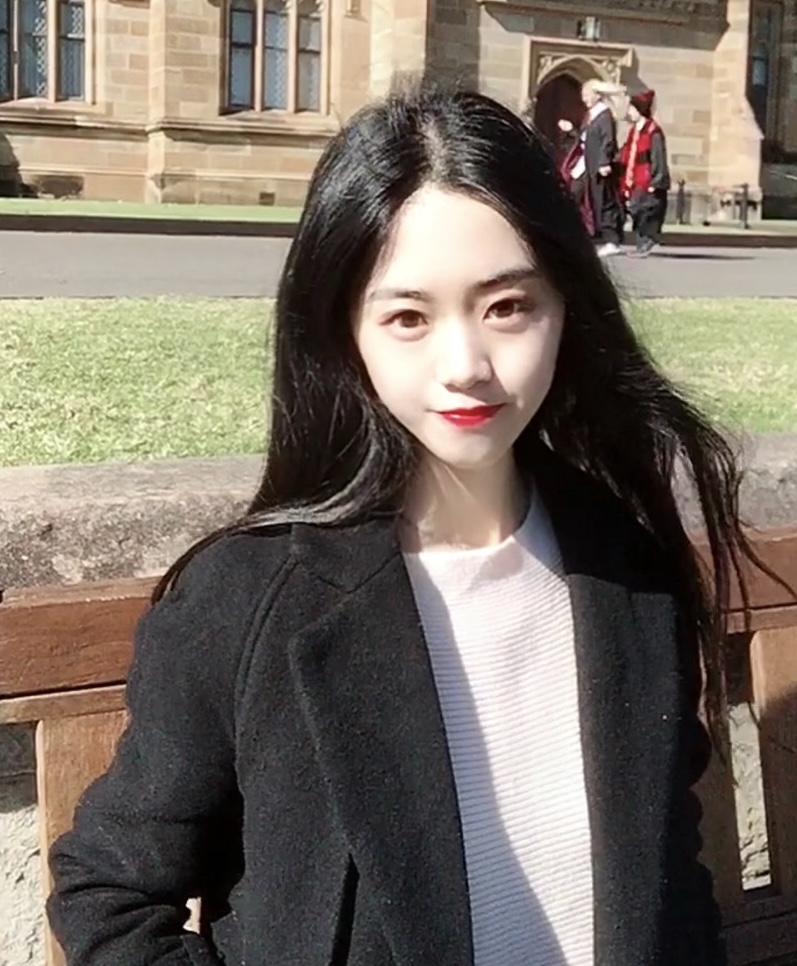
Yi Zhang
Contributed Members
Ruixiang Chen
Wanqi Yin
Qibin Yao
Keyu Chen
Mingyang Song
Zhongang Cai
Jingbo Wang
Zhengming Yu
Yang Gao
Zhengyu Lin
Daxuan Ren
Xiaopu Hao
Contact
We welcome contributions in the data and benchmarks of DNA-Rendering from the whole community! Interested in contributing or using DNA-Rendering, please feel free to drop an e-mail to Huiwen Luo (luohuiwen@pjlab.org.cn), and CC Wayne Wu (wuwenyan0503@gmail.com) and Kwan-Yee Lin (linjunyi9335@gmail.com).